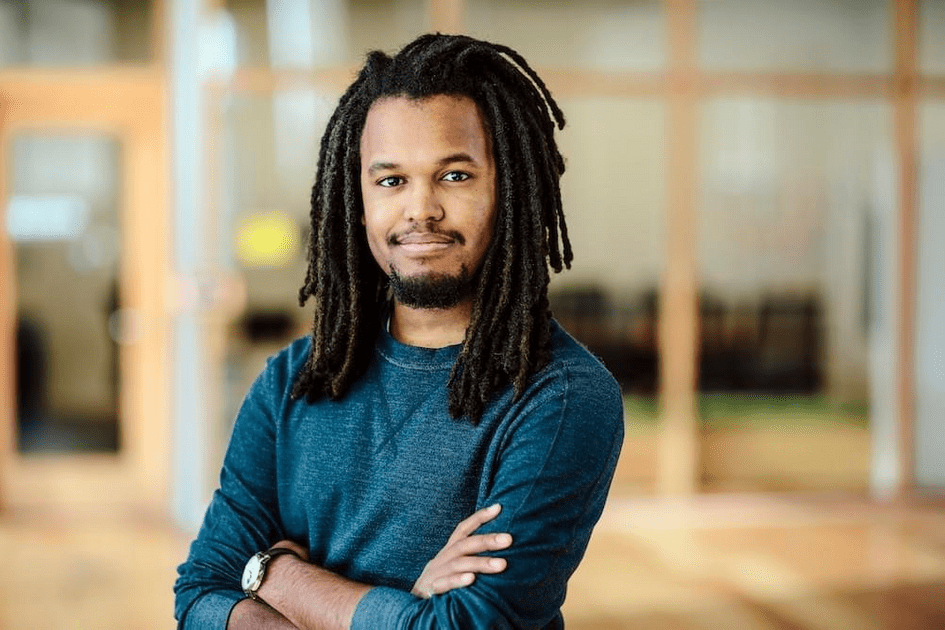
Yann N. Dauphin
Research Scientist
About
Yann N. Dauphin is a machine learning researcher at Google Research working on understanding the fundamentals of deep learning algorithms and leveraging that in various applications. He has published seminal work on understanding the loss surface of neural nets. Prior to joining Google in 2019, he was a researcher at Facebook AI Research from 2015 to 2018 where his work led to award-winning scientific publications and helped improve automatic translation on Facebook.com. He completed his PhD at U. of Montreal under the supervision of Prof. Yoshua Bengio. During this time, he and his team won international machine learning competitions such as the Unsupervised Transfer Learning Challenge in 2013.
Email: yann@dauphin.io Resume: Link
Research
Here are selected publications. Full list available on Google Scholar.
Published in Neural Information Processing Systems 2014
This paper dispels the myth of bad local minima in high dimension and shows that the loss surfaces of neural networks have remarkable properties. This seminal paper has helped renew interest for understanding non-convex optimization as well as the study of neural nets using methods from statistical physics.
Published in International Conference on Representation Learning 2017
Large deep neural networks are powerful, but exhibit undesirable behaviors such as memorization and sensitivity to adversarial examples. This work proposes a remarkably simple learning principle called mixup to alleviate these issues by encouraging the network to be linear between examples.
Published in International Conference on Machine Learning 2017
The prevalent approach to sequence to sequence problems such as translation was recurrent neural networks. In this work, we introduced a state-of-the-art architecture based entirely on convolutional neural networks. Compared to recurrent models, computations over all elements can be fully parallelized during training to better exploit the GPU hardware.
Awards
Here is a selected list of awards I've received.
Talks
© 2019